Read the new blog post by Lavinia Gambelli, Open Research Community Manager, about building an open research community at the University of Bristol on The Research Culture Blog.
Ethical Academic Publishing: How to Make Academic Publishing Fairer, More Open and Less Wasteful
By Dan Major-Smith
You find yourself on holiday in the strange and distant island of ‘El Seeveeyor’. As you walk through the narrow cobbled streets you chance upon a bustling market. At first glance all appears normal – farmers, traders and customers exchanging goods and gossiping about the humdrum of everyday life. But on closer inspection you notice something strange. The traders receive goods from the farmers, who in turn pass these over to customers; but both the farmers and customers ‘pay’ the traders for this service! “How could such a system exist?”, you think to yourself, “What do the farmers gain from this? And if the traders receive money from the farmers, why are the customers also paying? Is this an extortion racket?!”
We don’t need to travel to El Seeveeyor to witness this strange inversion in market logic – The same thing happens every day in academia. Researchers, funders and universities (‘farmers’, in the above scenario) – and ultimately the general public via taxes – pay for research to be conducted, while academic publishers (‘traders’) charge huge sums of money to both researchers to publish their research and readers (‘customers’) to access it.
Sure, the rather hackneyed analogy isn’t perfect, but nonetheless it hopefully gets across the fundamental strangeness of our academic publishing system. The point of this blog is not merely to point out its strangeness, but to demonstrate how it is fundamentally wasteful, needlessly expensive and discriminatory. In other words, it is unethical.
We should not blindly support this system, and should actively seek alternatives which are fairer, less costly and more inclusive. That is, we need a more ethical academic publishing system.
This blog is set out in three parts:
Part 1: The problem – describes the issues with the current academic publishing system;
Part 2: The cause – explores why the academic publishing system is structured this way; and
Part 3: The solution – discusses ways in which the academic publishing system can be altered to make it more ethical.
Part 1: The Problem
There are numerous issues with the current academic publishing system, two of the biggest being:
i) It is incredibly wasteful and does not represent value for money
The estimated profit margins of traditional ‘for-profit’ academic publishers (Elsevier, Springer, etc.) are enormous, at 30-50% (https://doi.org/10.1038/495426a). As an example, in 2010 Elsevier had approximately $2 (~£1.5) billion in revenue, of which $724 (~£550) million was profit – a profit margin of 36% (https://www.theguardian.com/science/2017/jun/27/profitable-business-scientific-publishing-bad-for-science; things have not changed much in the decade or so since then: https://www.ft.com/content/575f72a8-4eb2-4538-87a8-7652d67d499e). For reference, these profit margins rival other perceived ‘unethical’ businesses such as Apple, Google and Amazon, and are much higher than ‘standard’ business profit margins of around 10% (https://www.danielnettle.org.uk/2023/06/14/the-political-economy-of-scientific-publishing-and-the-promise-of-diamond-open-access/). Assuming a 30% profit margin, in 2017 the global revenue from academic publishing was ~$19 (~£14.5) billion, of which ~$6 (~£4.5) billion was pure profit which did not benefit science in any way (and presumably just filled shareholders’ pockets).
It has been estimated that it costs traditional publishers around £2,500 to publish a paper, on average. However, even after taking the extortionate profit margins into account, this likely exaggerates the costs, as it is realistically possible to publish a paper (and all associated costs such as journal maintenance) for approximately £300 (https://peercommunityin.org/2024/01/18/2023-finances-article-costs/; https://doi.org/10.7287/peerj.preprints.27809v1). While costs will of course vary by journal depending on various factors (e.g., dedicated/paid editors, proof-readers, production staff, etc.), essentially publishers convert a document to PDF, slap on some journal branding and upload it to a website. This should not cost nearly £10,000, to give a particularly egregious example (here’s looking at you, Nature Human Behaviour). Research has also found that published work is almost identical to pre-printed versions (https://doi.org/10.1007/s00799-018-0234-1), further questioning the ‘added value’ of these traditional academic publishers.
Large amounts of research funding money are therefore funnelled to academic publishers for seemingly little obvious benefit. A case study of Social Science disciplines in Austria, for instance, found that an estimated 25% of all publicly-funded research money ends up with academic publishers (https://doi.org/10.1371/journal.pone.0253226).
Rather than paying considerable sums of money – which, it bears repeating, greatly exceed the true costs of publishing – to simply publish and access research, this public money could be better spent funding more research, more permanent academic positions (especially for early-career researchers) or more support staff. Or this money could simply be spent elsewhere, such as addressing societal issues and supporting funding vital public services like the National Health Service.
ii) It results in discrimination and bias
Most forms of academic publishing result in bias and inequitable outcomes one way or another. Under the traditional ‘pay-to-read’ system (where publishing research is free but readers pay to access content) small institutions, charities, researchers unaffiliated with institutions and much of the global south are excluded from reading – and building on – research.
Conversely, under the newer ‘pay-to-publish’ system (where access is free but there is a cost to publishing), while those disadvantaged under the ‘pay-to-read’ system can now access research, they are unable to publish. This again leads to bias, this time in the scientific record.
(As an aside, this shift to the ‘pay-to-publish’ Open Access system has also led to perverse incentives for academic publishers operating under this model, as their income is simply a linear function of the numbers of papers published (https://doi.org/10.31219/osf.io/3ez9v). This can lead to lower quality research and editorial standards – e.g., https://www.vice.com/en/article/dy3jbz/scientific-journal-frontiers-publishes-ai-generated-rat-with-gigantic-penis-in-worrying-incident and https://deevybee.blogspot.com/2024/08/guest-post-my-experience-as-reviewer.html – and an increase in ‘predatory’ publishers).
Regardless of the publishing system, both models involve large (and, in the cause of university contracts with publishers, often undisclosed) costs to universities, funders and researchers, and prohibit the dissemination of, and access to, research; research which ought to be open and freely-available to all as it performs a public good (and, again, is largely funded by the public in the first place).
As a personal example, I have been fortunate to work at the University of Bristol, a Russell Group university, for the past few years, which has the resources to pay for subscriptions to (most) journals and Transformative Agreements to publish Open Access for free in many journals which would otherwise have article processing fees. In contrast, my wife has worked for both a conservation charity and a small university where it can be a struggle to both access articles and publish research Open Access due to the costs charged by academic publishers. My research is not more valuable than hers, yet the current academic publishing system creates and reinforces these inequalities.
Is this costly, wasteful and biased system really the best we can come up with as a research community? Surely we can design a fairer and more equitable publishing system which divests power from large publishers and provides more benefits for the research community? In the next section we’ll explore why this system persists.
Part 2: The Cause
Let’s start with two obvious premises: i) researchers need to publish; and ii) researchers respond to incentives (e.g., for jobs, promotion, funding, etc.). These factors interact and conspire to sustain the current academic publishing system. That is, researchers who publish in ‘prestigious’ or ‘high impact’ journals are more likely to be hired, promoted and receive funding.
Because of this, researchers, universities and funders will pay exorbitant article processing charges – even if they are almost completely divorced from the actual costs involved. In the fields of behavioural science and more ‘generalist’ scientific journals, there is an almost perfect correlation between a journal’s impact factor and their article processing costs (see figure below, taken from https://bsky.app/profile/sobchuk.bsky.social/post/3kggjewhicl2y). For a more detailed exploration of the factors influencing article processing fees, which finds similar patterns, see: https://doi.org/10.1162/qss_a_00015.
Despite this clear link, impact factor (and similar metrics of journal prestige) often have little – and sometimes potentially a negative! – relationship with measures of research quality and methodological rigour (https://doi.org/10.3389/fnhum.2018.00037).
The current system persists because of (perceived) journal prestige, which causes markets not to function as they normally would. Ordinarily a business making 40% profits would be outcompeted by a business charging less for the same product; but this ‘prestige’ effect means that markets do not work efficiently, especially as new journals – without established ‘prestige’ – are at a disadvantage.
In sum, the current system persists because we are paying for prestige, not the quality or value-for-money of the services provided (for a good historical overview of how this system emerged, see: https://www.theguardian.com/science/2017/jun/27/profitable-business-scientific-publishing-bad-for-science).
How do we overcome this? And what changes could make the publishing system more ethical?
Part 3: The Solution
There are a number of solutions to make academic publishing more ethical, operating at a range of different levels.
At the broad cultural level, it is clear that we need to shift away from metrics such as journal prestige, impact factors and number of publications, and focus on research quality. While somewhat nebulous, this includes factors such as: methodological rigour and scientific integrity; open science practices such as preregistration/Registered Reports and sharing data/code/materials to facilitate reproducible and replicable research; and a focus on team – rather than solely individual – contributions to research (https://doi.org/10.1177/17456916231182568).
This is of course easier said than done, especially with the current incentive structure still largely in place regarding academic promotions and funding. Part of this change has to be top-down. That is, institutions need to stop hiring and promoting, and funding bodies need to stop funding, academics based on perceived prestige – e.g., having Nature, Science or Cell publications – and instead focus on research quality. Many such guidelines and principles have been suggested (e.g., https://doi.org/10.1371/journal.pbio.3000737 and https://doi.org/10.1371/journal.pbio.2004089), and are increasingly being developed and put into practice, such as the Declaration on Research Assessment (DORA), the ‘Recognition and Rewards’ programme in the Netherlands and the ‘Open and Responsible Researcher Reward and Recognition’ (OR4) programme in the UK.
This top-down institutional-level change is likely to be slow, however, so bottom-up pressure directly from researchers is also needed. A number of options are available (for overviews, see https://doi.org/10.12688/f1000research.11415.2 and https://doi.org/10.1111/ele.14395):
- Ignore (or give less weight to) journal prestige and impact factor when deciding where to publish. This strategy may be potentially risky, especially for early-career researchers, but is ultimately necessary. Given these potential risks, established academics with permanent contracts need to lead the way on this. As institutions are beginning to focus more on research quality in hiring and promotion decisions – the University of Bristol have signed up to DORA and are part of the OR4 programme, for instance – this strategy should become less risky with time.
- Preferentially publish in Open Access ‘not-for-profit’ journals, which invest any profits back into academia. This includes Diamond Open Access journals (which are free to both publish in and access; e.g., ‘Peer Community In’, ‘PsychOpen Gold’ or ‘Open Library of Humanities’) and other ‘not-for-profit’ Open Access journals (which charge article processing fees; e.g., PLOS or eLife). For a list of Diamond Open Access journals, see: https://zenodo.org/records/4562828.
- If you do publish in a ‘pay-to-read’ journal (or in a hybrid journal and cannot pay the Open Access Article Processing Charges), upload a preprint and/or the author-accepted manuscript to a relevant Open Access repository (e.g., OSF, arXiv, medRxiv, bioRxiv, PsyArXiv, or an institutional repository). This is known as ‘green’ Open Access).
- Use tools such as those recently developed by Plan S (an Open Access publishing initiative) to estimate how ‘equitable’ (i.e., ethical) a scholarly communication (e.g., publishing in a given journal) is (https://www.coalition-s.org/new-tool-to-assess-equity-in-scholarly-communication-models/).
- Avoid publishing (or publish less) in traditional ‘for-profit’ journals (e.g., those run by Elsevier, Wiley, Sage, Springer, Taylor & Francis, Frontiers, etc.).
- Preferentially review and act as an editor for these more ‘ethical’ not-for-profit journals to help increase support and growth of these journals (e.g., Peer Community In: Registered Reports are currently looking for editors/recommenders).
- If on an editorial board of a for-profit journal, consider encouraging them to reduce their publication fees or threaten to resign from your position (http://corinalogan.com/journals.html). Many editorial boards have walked out en masse for these reasons (e.g., the Elsevier-owned journal ‘Neuroimage’; https://www.theguardian.com/science/2023/may/07/too-greedy-mass-walkout-at-global-science-journal-over-unethical-fees).
- Set up an alternative ethical journal. Again, many editorial boards have done this (see Neuroimage above, and also https://www.theguardian.com/commentisfree/article/2024/jul/16/academic-journal-publishers-universities-price-subscriptions). There is often infrastructure in place to do this relatively easily (e.g., setting up a ‘Peer Community In’ journal for a new discipline).
- Lobby your institution to develop and implement clear promotion guidelines which rest on research quality.
- University libraries can choose to help fund and support grassroots Diamond Open Access journals such as Peer Community In and Open Library of Humanities (both of which, and other similar initiatives, are supported by the University of Bristol, among numerous other institutions).
- Libraries can also not renew – or negotiate better and more ethical – contracts with for-profit publishers, as occurred in Germany, the University of California and MIT.
- Finally, spread the word about unethical publishing and how to overcome it to colleagues. Many researchers are aware of the issues with the current system, but there is little coordination for collective action to occur. Discussing these concerns with others is a good start.
Change will likely be slow, and traditional for-profit publishers will naturally push back on these changes (or purchase these fledgling ‘not-for-profit’ journals/publishers). But ultimately it is incumbent on researchers to be the change they want to see. For me personally, while I may still publish in traditional ‘for-profit’ journals occasionally, I try and support Diamond Open Access and not-for-profit publishers more where possible. But as an early/mid-career researcher, there is only so much impact I can have. There are many unknowns, and lots I am still learning about the academic publishing system. I should also note that my perspective is largely from a STEM background and mainly concerns journal article publishing; while there are some small differences which it comes to Arts, Humanities and Social Science disciplines (e.g., a greater focus on books, which can be more difficult to publish Open Access), the overall picture is broadly similar.
Pulling all this together, I have found the following image a useful roadmap when thinking about how to publish ethically (although whether there is a single dimension from ‘least ethical’ to ‘most ethical’ is perhaps a dubious assumption, and there will also be journal-level variation within publishers) – I hope it may help you too.
I definitely do not have all the answers, but these conversations are certainly worth having. If we can make the academic publishing system fairer, less wasteful and more ethical, then I believe we need to try.
Author:
Dan Major-Smith is a somewhat-lapsed Evolutionary Anthropologist who now spends most of his time working as an Epidemiologist. He is currently a Senior Research Associate in Population Health Sciences at the University of Bristol, and works on various topics, including selection bias, life course epidemiology and relations between religion, health and behaviour. He is also interested in meta-science/open scholarship more broadly, including the use of pre-registration/Registered Reports, synthetic data and ethical publishing. Outside of academia, he fosters cats and potters around his garden pleading with his vegetables to grow.
Our new policy on open research (from the Research Culture blog)
Read the new blog post by Marcus Munafò, Associate Pro Vice-Chancellor – Research Culture, about our new policy on open research on The Research Culture Blog.
The Seven Sins of Experimental Design and Statistics
By Michelle Taylor
Reproducibility is fast becoming the ‘currency’ of credible, robust, scientific research. If a result cannot be reproduced with the same dataset or methods, then it questions the validity of the original work. I am the resident Biostatistician in the Life and Health Sciences Faculty, and it is my job to ensure that biological research is reproducible, so that the University of Bristol fulfils its legal obligations in terms of the 3Rs of animal welfare, and maintains its credible reputation. Here I describe seven reproducibility ‘sins’ that I commonly encounter along with some solutions to help researchers improve the reproducibility of their work. They cover the experimental journey from design, to analysis, and final publication.
The Seven Sins of Experimental Design and Statistics
Experimental Design
1. Insufficient knowledge about the sample population
The first ‘sin’ is an insufficient knowledge of the sample population. When we take a sample of data, we need to ensure that it is suitably random and representative of the population we want to study. This means we need to understand much more about where the experimental samples have come from before they end up in the experiment. For example, zebrafish are cultured under a wide range of environmental parameters, which means that the effects produced in different labs could have been influenced by more than just the experimental treatment. Unknown sources of variation contribute to a lack of reproducibility between studies conducted at various times and places. The solution is to understand as much as possible about where the samples come from so that all relevant sources of variation can be identified and controlled.
2. Lack of randomization and blinding
The second ‘sin’ is inappropriate randomization and/or blinding. Randomizing treatments to independent samples and preventing the researcher from knowing these details at the time of data collection, is the primary method of accounting for sources of bias during an experiment. Biases contribute to a lack of reproducibility as they obscure the true treatment effects and weaken the validity of the experiment. The solution is to think carefully about which experimental units should be randomly allocated, and also how, when, and by whom to avoid biases in procedure, time, and personnel.
Statistical Analysis
3. Inappropriate analysis
The next ‘sin’ is inappropriate analysis for the data. All datasets have a set of characteristics, such as the type of outcome that is measured, the number of variables that are used to explain the outcome, and other structural features that provide context. There are numerous statistical approaches available, but unfortunately, too often an experiment is reduced to a relatively simple analysis, such as a t-test or Chi-sq. This is not only incorrect use of statistical tests but is also a missed opportunity to explore a biological question in greater context. The solution is for researchers to upgrade and expand their statistical knowledge to be able to apply the best analysis to match the complexity of the experiment.
4. Pseudoreplication
A relatively common ‘sin’ is pseudoreplication. This is simply either a lack of adequate replication of independent experimental units or incorrect application of statistical tests to the correct unit of replication. The most common source of pseudoreplication involves using a test that was intended for independent samples on data derived from clustered samples. A classic example is when ten mice are measured three times each in an experiment, and all 30 datapoints are put into a t-test. The repeated measurements of each mouse mean than the standard error for the mean of the group is too small, relative to that of a random, independent sample of 30 mice. This makes it more likely that the t-test gives a spuriously small p value that cannot be replicated in another study using the correct sample size of ten. The solution is to understand the correct unit of replication for the hypothesis of interest. Multiple measurements of the same unit require tests of related datapoints, such as repeated measures ANOVA or longitudinal analyses. Similarly, samples that come from a clear hierarchical structure – littermates, tanks of fish, plots of land within a field – require tests that take account of the clear nesting of treatment groups within a larger structure.
5. Insufficient power and overreliance on p values
The final analysis ‘sin’ is insufficient statistical power and overreliance on p values to determine whether a treatment had an effect. Low statistical power and small p values contribute to a lack of reproducibility because they themselves are unreliable. The solution is to understand the relationship between N (total sample size), the effect size, and statistical power. The most essential element in any analysis is the effect size – the magnitude of difference between treatment groups. Statistical power is the ability of the statistical test to ‘see’ the effect size. Large sample sizes provide statistical power by reducing the width of the confidence intervals around the group means, making effect size differences more obvious. At the correct sample size, all effect size differences will break the threshold of significance in a statistical test. The conventional aim for a study is to have statistical power of 0.8, or 80% chance of finding the significant effect an experiment is designed to produce, and there are many tools available to help researchers find the correct sample size to achieve this.
Interpretation / Reporting
6. Overinterpreting the data
The next ‘sin’ is overinterpreting the data. We have an established hierarchy of evidence in biosciences, where observational studies rank lower in their ability to convince the reader than experimental studies, which rank lower than reviews and meta-analysis. When chance findings and highly exploratory work are presented as if they were more rigorously designed confirmatory tests of a specific hypothesis, or claims are made using data that was highly specific or restricted in the samples used, results are difficult to reproduce. This is because the foundations of the original claims were not as high up the hierarchy as stated. The solution is to be clear about the limitations of the experiment, acknowledge sources of bias, and realize what the data show. Reproducible science does not mean simply finding the same things again, but being transparent and realistic in what was done and the limits of what we can claim.
7. Insufficient detail to correctly interpret the results
Finally, the most important ‘sin’ of all – insufficient detail to correctly interpret the results. Large scale reviews undertaken by major scientific governing bodies in the UK and US have shown that most of the failure to reproduce results – that is, to directly repeat the finding of a previous study using the same data – was due to a lack of sufficient information to repeat the experiment or analysis. Key areas that are historically and routinely missing from research papers include randomization and blinding, sample size calculations, statistical details, effect sizes, and details of the housing and husbandry of the study population. The solution is to increase publishing standards to improve communication. To achieve this, there is a growing collection of guidelines, such as ARRIVE, TOP and SAMPL, which can be used to standardize publishing requirements and ensure experiments are faithfully reported and can be reproduced.
~~~~
Tackling reproducibility and demonstrating that scientific methods and research are robust is becoming a key determinant of funding success, ethical approval, and publishing, so it is in all our interests to be aware of these issues and their solutions.
Resources and further information:
Webpages on the staff intranet for Life & Health Sciences: https://uob.sharepoint.com/sites/life-sciences/SitePages/Reproducibility-in-Life-Sciences.aspx
Altman DG. 1982. How large a sample? In: Gore SM AD, editor. Statistics in Practice l. London, UK: British Medical Association https://doi.org/10.1136/bmj.281.6251.1336
Serdar, C.C. et al. 2021. Sample size, power and effect size revisited: simplified and practical approaches in pre-clinical, clinical and laboratory studies. Biochem Med, 31(1): 010502. https://doi.org/10.11613/BM.2021.010502
HoC Science, Innovation and Technology Committee. Reproducibility and Research Integrity. 2023. https://publications.parliament.uk/pa/cm5803/cmselect/cmsctech/101/report.html
Hurlbert, S.H. 1984. Pseudoreplication and the Design of Ecological Field Experiments. Ecological Monographs, 54, 187-211. https://doi.org/10.2307/1942661
Lazic, S.E., 2010. The problem of pseudoreplication in neuroscientific studies: is it affecting your analysis? BMC Neuroscience, 11:5. https://doi.org/10.1186/1471-2202-11-5
Lazic, S.E., et al. 2018. What exactly is ‘N’ in cell culture and animal experiments? PLoS Biology. 16:e2005282. https://doi.org/10.1371/journal.pbio.2005282
National Academies of Sciences, Engineering, and Medicine. (2019). Reproducibility and Replicability in Science. Washington, DC: The National Academies Press. https://doi.org/10.17226/25303
Taylor, M. (2024). Experimental Design for Reproducible Science. Talk given at Reproducibility by Design symposium, University of Bristol, June 2024. https://doi.org/10.17605/OSF.IO/NCWE6
Author:
Dr Michelle Taylor is a Senior Research Associate in Statistics and Experimental Design based at the University of Bristol. Her research background is in Evolutionary Genetics and Ecology, focusing on sexual selection and reproductive fitness, and she has previously held research positions at the Universities of Exeter and Western Australia. She moved into her current role at University of Bristol in 2018 to support the Animal Welfare Ethics Review Board and promote a wider focus on reproducibility across the Biosciences.
AI-based tools to support research data management
As datasets grow in size and complexity, traditional methods of data management may be insufficient to meet the demands of modern research. This is where AI-based tools can come into play, offering a suite of powerful capabilities to streamline and enhance data management processes. These tools are designed to address a variety of challenges that researchers face, from data collection and cleaning to storage, analysis, and security. The integration of AI in research data management can enable researchers to focus on higher-level like tasks such as hypothesis generation and theory building, whilst helping maintain scientific reproducibility. But despite these advantages, it is important to recognise that AI tools are not a panacea, and present both opportunities and threats to open research. For example, they require careful selection and implementation to address specific research needs, and the reliance on AI necessitates a degree of proficiency in data science, which might be a barrier for some researchers. There can also be concerns over data reuse, and questions about the motivations of major-league software developers. Nonetheless, we’ve noticed some AI-based software tools that seem to be achieving prominence. Below is a list of these. They are not intended as recommendations, but may provide a starting point for critical evaluation.
Finally, an interesting perspective on the use of AI in science was provided by UoB’s Pen-Yuan Hsing in his recent talk at the Reproducibility by Design symposium in Bristol on 26th June: “AI is not the problem – thinking about outcomes”.
Data Collection and Integration
Google Data Studio allows researchers to turn data into informative, easy-to-read, shareable, and customisable dashboards and reports. Its AI capabilities help integrate and visualize data from multiple sources.
Keboola leverages AI to integrate various data sources, automate workflows, and ensure data consistency, aiding researchers in managing complex datasets.
Data Cleaning and Preparation
Trifacta uses AI to simplify data wrangling, helping researchers clean and prepare their data for analysis. It identifies patterns and anomalies.
Talend provides AI-powered data integration and data integrity solutions, allowing researchers to clean, transform, and govern data efficiently.
Data Storage and Management
Datalore is an AI-driven collaborative data science platform that allows researchers to create, run, and share Jupyter notebooks in the cloud.
Azure Data Lake provides a scalable and secure data storage solution, with AI capabilities to manage large datasets and perform big data analytics.
RapidMiner uses AI to facilitate data mining, machine learning, and predictive analytics. It offers a visual workflow designer for data preparation, model building, and evaluation.
KNIME Analytics Platform is an open-source software that integrates various components for machine learning and data mining through a modular data pipelining.
Using Synthetic Datasets to Promote Research Reproducibility and Transparency
By Dan Major-Smith
Scientific best practice is moving towards increased openness, reproducibility and transparency, with data and analysis code increasingly being publicly available alongside published research. In conjunction with other changes to the traditional academic system – including pre-registration/Registered Reports, better research methods and statistics training, and altering the current academic incentive structures – these shifts are intended to improve trust, reproducibility and rigour in science.
Making data and code openly available can improve trust and transparency in research by allowing others to replicate and interrogate published results. This means that the published results can be independently verified, and can even help spot potential errors in analyses such as in this and this high-profile examples. In these cases, because data and code were open, errors could be spotted and the scientific record corrected. It is impossible to know how many papers without associated publicly available data and/or code suffer from similar issues. Because of this, journals are increasingly mandating both the data and code sharing, with the BMJ being a recent example. As another bonus, if data and code are available, readers can test out potentially new analysis methods, improving statistical literacy.
Despite these benefits and the continued push towards data sharing, many researchers still do not openly share their data. While this varies by discipline, with predominantly experimental fields such as Psychology having higher rates of data sharing, there is plenty of room for improvement. In the Medical and Health Sciences, for instance, a recent meta-analysis estimated that only 8% of research was declared as ‘publicly available’, with only 2% actually being publicly available. The rate of code sharing was even more dire, with less than 0.5% of papers publicly sharing analysis scripts.
~~~~
Although data sharing should be encouraged wherever possible, there are some circumstances where the raw data simply cannot be made publicly available (although usually the analysis code can). For instance, many studies – and in particular longitudinal population-based studies which collect large amounts of data on large numbers of people for long periods of time – prohibit data sharing for reasons of preserving participant anonymity and confidentiality, data sensitivity, and to ensure that only legitimate researchers are able to access the data.
ALSPAC (the Avon Longitudinal Study of Parents and Children; https://www.bristol.ac.uk/alspac/), a longitudinal Bristol-based birth cohort housed within the University of Bristol, is one such example. As ALSPAC has data on approximately 15,000 mothers, their partners and their offspring, with over 100,000 variables in total (excluding genomics and other ‘-omics’ data), it has a policy of not allowing data to be released alongside published articles.
These are valid reasons for restricting data sharing, but nonetheless are difficult to square with open science best practices of data sharing. So, if we want to share these kinds of data, what can we do?
~~~~
One potential solution, which we have recently embedded within ALSPAC, is to release synthetic data, rather than the actual raw data. Synthetic data are modelled on the observed data which maintain both the original distributions of the data (e.g., means, standard deviations, cell counts) and the relationships between variables (e.g., correlations between variables). Importantly, while maintaining the key features of the original data, the data are generated from statistical models, meaning that observations do not correspond to real-life individuals, hence preserving participant anonymity.
These synthetic datasets can then be made publicly available alongside the published paper in lieu of the original data, allowing researchers to:
- Explore the raw (synthetic) data
- Understand the analyses better
- Reproduce analyses themselves
A description of the way in which we generated synthetic data for our work is included in the ‘In depth’ section at the end of this blog post.
While the synthetic data will not be exactly the same as the observed data, making synthetic data openly available does add a further level of openness, accountability and transparency where previously no data would have been available. Further, synthetic datasets can provide a reasonable compromise between the competing demands of promoting data sharing and open-science practices while maintaining control over access to potentially sensitive data.
Given these features, working with the ALSPAC team, we developed a checklist for generating synthetic ALSPAC data. We hope that users of ALSPAC data – and researchers using other datasets which currently prohibit data sharing – make use of this synthetic data approach to help improve research reproducibility and transparency.
So, in short: Share your data! (but if you can’t, share synthetic data).
~~~~
Reference:
Major-Smith et al. (2024). Releasing synthetic data from the Avon Longitudinal Study of Parents and Children (ALSPAC): Guidelines and applied examples. Wellcome Open Research, 9, 57. DOI: 10.12688/wellcomeopenres.20530.1 – Further details (including references therein) on this approach, specifically applied to releasing synthetic ALSPAC data.
Other resources:
The University Library Services’ guide to data sharing.
The ALSPAC guide to publishing research data, including the ALSPAC synthetic data checklist.
The FAIR data principles – there is a wider trend in funder, publisher and institutional policies towards FAIR data, which may or may not be fully open but which are nevertheless accessible even where circumstances may prevent fully open publication.
Author:
Dan Major-Smith is a somewhat-lapsed Evolutionary Anthropologist who now spends most of his time working as an Epidemiologist. He is currently a Senior Research Associate in Population Health Sciences at the University of Bristol, and works on various topics, including selection bias, life course epidemiology and relations between religion, health and behaviour. He is also interested in meta-science/open scholarship more broadly, including the use of pre-registration/Registered Reports, synthetic data and ethical publishing. Outside of academia, he fosters cats and potters around his garden pleading with his vegetables to grow.
~~~~
In depth
In our recent paper, we demonstrate how synthetic data generation methods can be applied using the excellent ‘synthpop’ package in the R programming language. Our example is based on an openly available subset of the ALSPAC data, so that researchers can fully replicate these analyses (with scripts available on a GitHub page).
There are four main steps when synthesising data, which we demonstrate below, along with example R code (for full details see the paper and associated scripts):
1. After preparing the dataset, create a synthetic dataset, using a seed so that results are reproducible (here we are just using the default ‘classification and regression tree’ method; see the ‘synthpop’ package and documentation for more information)
dat_syn <- syn(dat, seed = 13327)
2. To minimise the potential disclosure risk, when synthesising ALSPAC data we recommend removing individuals who are uniquely-identified in both the observed and synthetic datasets (in this example, only 4 of the 3,727 observations were removed [0.11%])
dat_syn <- sdc(dat_syn, dat, rm.replicated.uniques = TRUE)
3. Compare the variable distributions between the observed and synthetic data to ensure these are similar (see image below)
compare(dat_syn, dat, stat = “count”)
4. Compare the relationships between variables in the observed and synthetic data to check similarity, here using a multivariable logistic regression model to explore whether maternal postnatal depressive symptoms are associated with offspring depression in adolescence (see image below)
model.syn <- glm.synds(depression_17 ~ mat_dep + matage + ethnic + gender + mated + housing, family = “binomial”, data = dat_syn) compare(model.syn, dat)
As can be seen, although there are some minor differences between the observed and synthetic data, overall the correspondence is quite high.
GW4 Open Research Prize 2023: Theory of Change (from the Research Culture blog)
Read the new blog post by Christopher Warren, Assistant Research Support Librarian, about the GW4 Open Research Prize 2023 on The Research Culture Blog.
A Scholarly Works Policy for the University of Bristol
A new Scholarly Works Policy was approved at the April meeting of Senate. Here we set out the reasons for the policy, what it does, and how it will work.
Why are we introducing this policy?
The University is committed to improving research culture and – as part of this – supporting and enabling open research practices. The ability to publish our research Open access, ensuring free and unrestricted access to research outputs, is an essential part of this. Open Access has also become an expectation of research assessment exercises such as the REF, as well as a requirement of many funders (including UKRI and Wellcome).
Gold Open Access (paying publishers to publish the “version of record” Open Access via Article Processing Charges (APCs) and “transformative agreements”) is well established in many disciplines, but now green Open Access (self-archiving the author manuscript in an institutional repository) is becoming increasingly common.
The development of a robust green route to Open Access publishing promotes an inclusive research culture by making Open Access publishing available to all, regardless of academic position and current funding, and mitigates the risks of choosing to publish Open Access for individual researchers when navigating a complex publishing landscape. With most Russell Group Institutions implementing similar policies, it also strengthens our collective hand when negotiating with publishers for Open Access services.
The University’s new Scholarly Works policy uses the concept of “rights retention” to support authors in choosing to self-archive. With Rights Retention, authors can disseminate their work as widely as possible while also meeting funder and any future REF requirements.
What is rights retention?
Traditionally, publishers require that authors sign a Copyright Transfer Agreement. The only way to access the article after publication is to pay for it. Rights Retention is based on the simple principle that authors and institutions should retain some rights to their publications.
The policy provides a route for researchers to deposit their author accepted manuscript in our institutional repository, and, using a rights retention statement, both retain the rights within their work, and grant the University a licence to make the author accepted manuscript of their scholarly article publicly available under the terms of a Creative Commons Attribution (CC BY) licence.
What does this mean for researchers?
This policy should not involve a major increase in administrative burden for researchers. There will be very little change to researcher workflows – in fact, as part of the review of workflows Library Services is undertaking, there will be a reduction in the number of steps required for Pure submissions in many cases.
Library Services will be updating their webpages, guidance, training and instructional videos so that researchers can feel confident about using this policy. If you have questions, comments or feedback, please get in touch because it could be helpful in shaping this guidance. You can contact us by emailing lib-research-support@bristol.ac.uk
The Uncertain Space: a virtual museum for the University of Bristol
The Uncertain Space is the new virtual museum for the University of Bristol. It is the result of a joint project between Library Research Support and Cultural Collections, funded by the AHRC through the Capability for Collections Impact Funding, which also helped fund the first exhibition.
The project originated in a desire to widen the audience to some of the University’s collections, but in a sustainable way which would persist beyond the end of the project. Consequently, The Uncertain Space is a permanent museum space with a rolling programme of exhibitions and a governance structure, just like a physical museum.
The project had two main outcomes: the first was the virtual museum space and the second was the first exhibition to be hosted in the museum. The exhibition, Secret Gardens, was co-curated with a group of young Bristolians, aged 11-18 and explores connections between the University’s public artworks and some of the objects held in our rich collections.
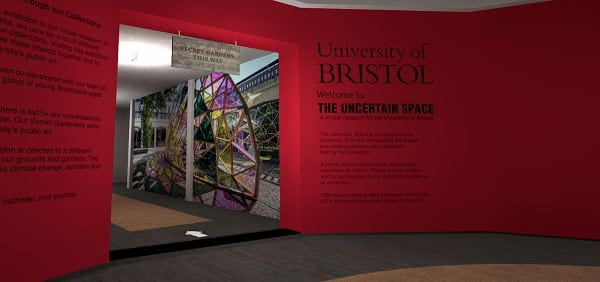
The group of young people attended a series of in-person and online workshops to discover their shared interests and develop the exhibition. The themes of identity, activism and environmental awareness came through strongly and these helped to inform their choice of items for the exhibition.
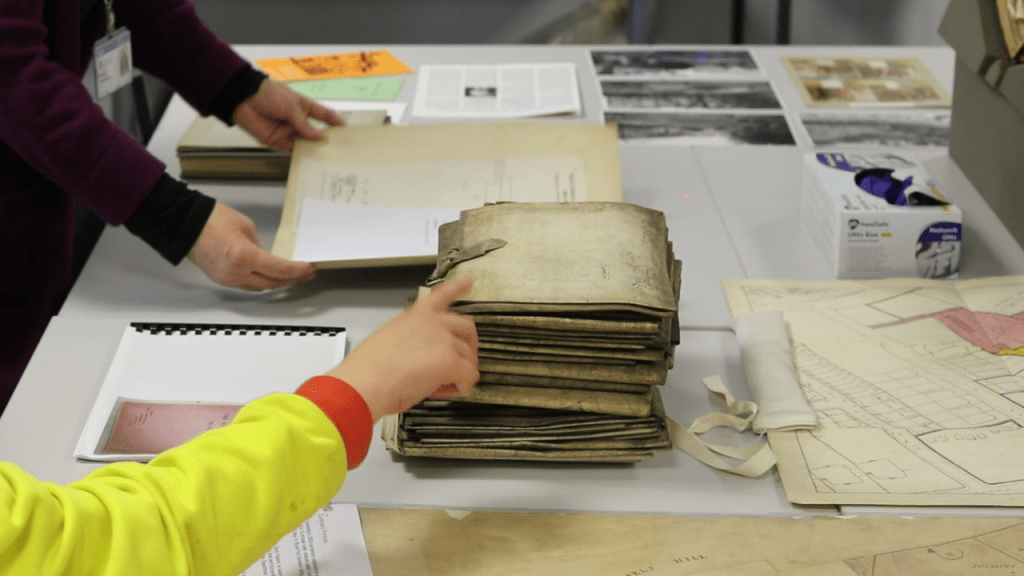
Objects, images and audiovisual clips, to link with each of the public artworks, were selected from the Theatre Collection, Special Collections, the Botanic Gardens and from collections held in the Anatomy, Archaeology and Earth Sciences departments. For some of the choices, digital copies already existed, but most of the items had to be digitised by photography or by scanning, using a handheld structured light scanner. The nine public artworks were captured by 360 degree photography. In addition, the reactions of the young people were recorded as they visited each of the public artworks and these are also included in the exhibition.
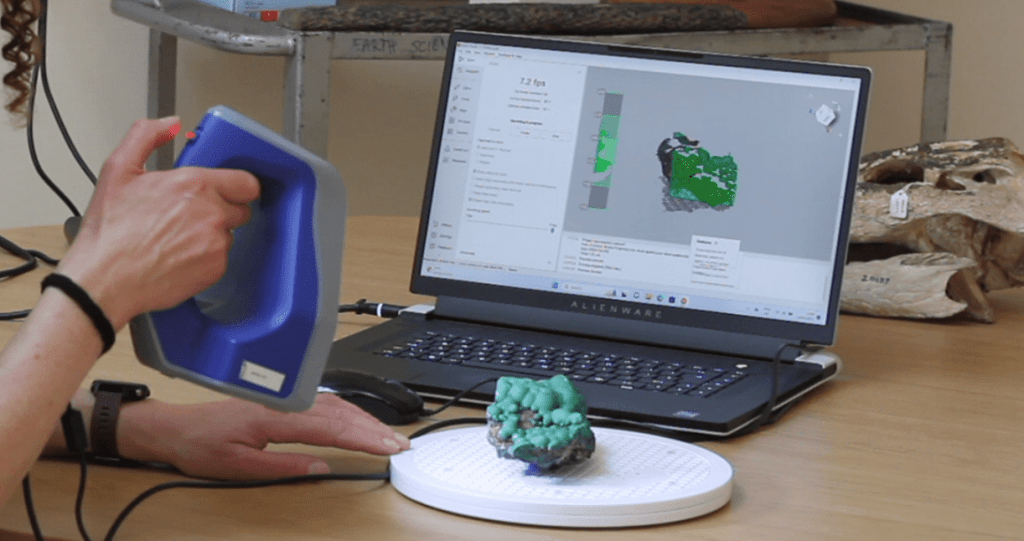
As the virtual museum was designed to mimic a real-world exhibition, the University of Bristol team and the young people worked with a real-world exhibition designer, and it was found that designing a virtual exhibition was a similar process to designing a real-world exhibition. Some aspects of the process, however, were unique to creating a virtual exhibition, such as the challenges of making digital versions of some objects. The virtual museum also provides possibilities that the real-world version cannot, for example the opportunity to pick up and handle objects and to be transported to different locations.
Towards the end of the project, a second group of young people, who were studying a digital music course at Creative Youth Network, visited the virtual museum in its test phase and created their own pieces of music in response. Some of these are included in a video about the making of the museum.
The museum and first exhibition can be visited on a laptop, PC or mobile device via The Uncertain Space webpage, by downloading the spatial.io app onto a phone or VR headset, or by booking a visit to the Theatre Collection or Special Collections, where VR headsets are available for anyone to view the exhibition.
We are looking forward to a programme of different exhibitions to be hosted in The Uncertain Space and are interested in hearing from anyone who would like to put on a show.
You can read more about the making of The Uncertain Space and its first exhibition from our colleagues in Special Collections and Theatre Collection:
Our collections go virtual!
Digitising for the new virtual museum: The Uncertain Space
Case Study: Library Research Metrics Service
This is the first of a series of qualitative case studies exploring the work and impact of Library Research Support activities and services. This case study focuses on the Library Research Metrics Service.
What we do
The Library Research Metrics Service provides support to individuals with research metrics queries, via training on a range of research metrics platforms, and education and outreach to ensure the university’s commitments to responsible use of research metrics are upheld. This is designed to complement support offered by the Department of Research, Enterprise and Innovation’s Research Information and Evaluation team which has a wider remit covering strategic research intelligence and support for large grant bids.
As well as an email enquiry service and web guidance, the Library Research Metrics Service provides training via online workshops, open to all academics and postgraduate researchers. These serve as an introduction to the concept of citation metrics and alternative metrics, what they can and cannot be used for, the principles of responsible metrics, and the importance of data accuracy – including how this may be improved through the use of ORCID researcher identifiers. Sessions also include live demonstrations on the tool, platform, or process of attendees’ choice: for example, how to create bespoke reports in SciVal, how to find alternative metrics in Scopus or Altmetric Explorer, or how to clean up author profiles in Scopus and other bibliographic databases.
Outreach activities are a key part of the support service; currently the ORCID promotion campaign is the main focus for outreach activities. This campaign seeks to increase ORCID signup rates among research staff and PGRs, which with support from Faculty Research Directors will be achieved in a variety of ways:
- Direct communication with the small subset of researchers that have an ORCID but have not fully synchronised it to their Pure profile
- Talks at School assemblies and other relevant gatherings
- PGR-led promotion activities
- Passive communication via posters and banners in key locations
- Active encouragement via a prize draw for new ORCID signups
Enquiry types
The email enquiry service receives a range of enquiry types: primarily these relate to 1) use of specific metrics platforms, 2) requests for metric support for grant for promotion bids, 3) queries about the use of metrics to support decisions on journal choice. Often a large part of the response to these enquiries is educational rather than direct provision of the resources requested. For example, both DORA and the University’s own statement on Responsible Research Evaluation state that research outputs must be considered on their own merits rather than the reputation or ranking of the journal or publisher. Therefore, a significant part of enquiry work is responding sensitively to researchers with these types of queries, to explain why metrics may not necessarily be helpful in making these decisions and to signpost to alternative tools and methods for journal selection. There are some instances where specific metrics can be useful: for example, establishing proportions of article types published in a given journal to identify titles most likely to be receptive to submission of similar manuscripts. In these instances, the Library Research Metrics Service will demonstrate how these metrics can be obtained or provide bespoke reports.
Another common query category comes from researchers who are finding unexpected results when seeking metrics data on their own publications: typically, missing publications or missing citations. Support in these instances usually takes two formats: 1) an investigation into and explanation of any data inaccuracies and suggestions for how these may be addressed, and 2) education on the limitations of metrics platforms – which is particularly relevant for researchers working in disciplines that are not covered well by the main bibliometrics platforms (arts, humanities, and those working in languages other than English, to name a few).
Outcomes and next steps
Responses to these education and outreach activities have largely been positive, with researchers praising the service for providing “really helpful” information. Certain departments or units are frequent flyers to the service – for example ALSPAC – but generally users tend to have a single query only. It remains to be seen whether the raised profile of the Library Metrics Service provided by the ORCID promotion campaign will result in larger volume of enquiries. In future, workshops will be run in person as well, and online workshops will be provided asynchronously to enable wider uptake.